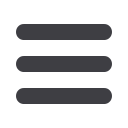
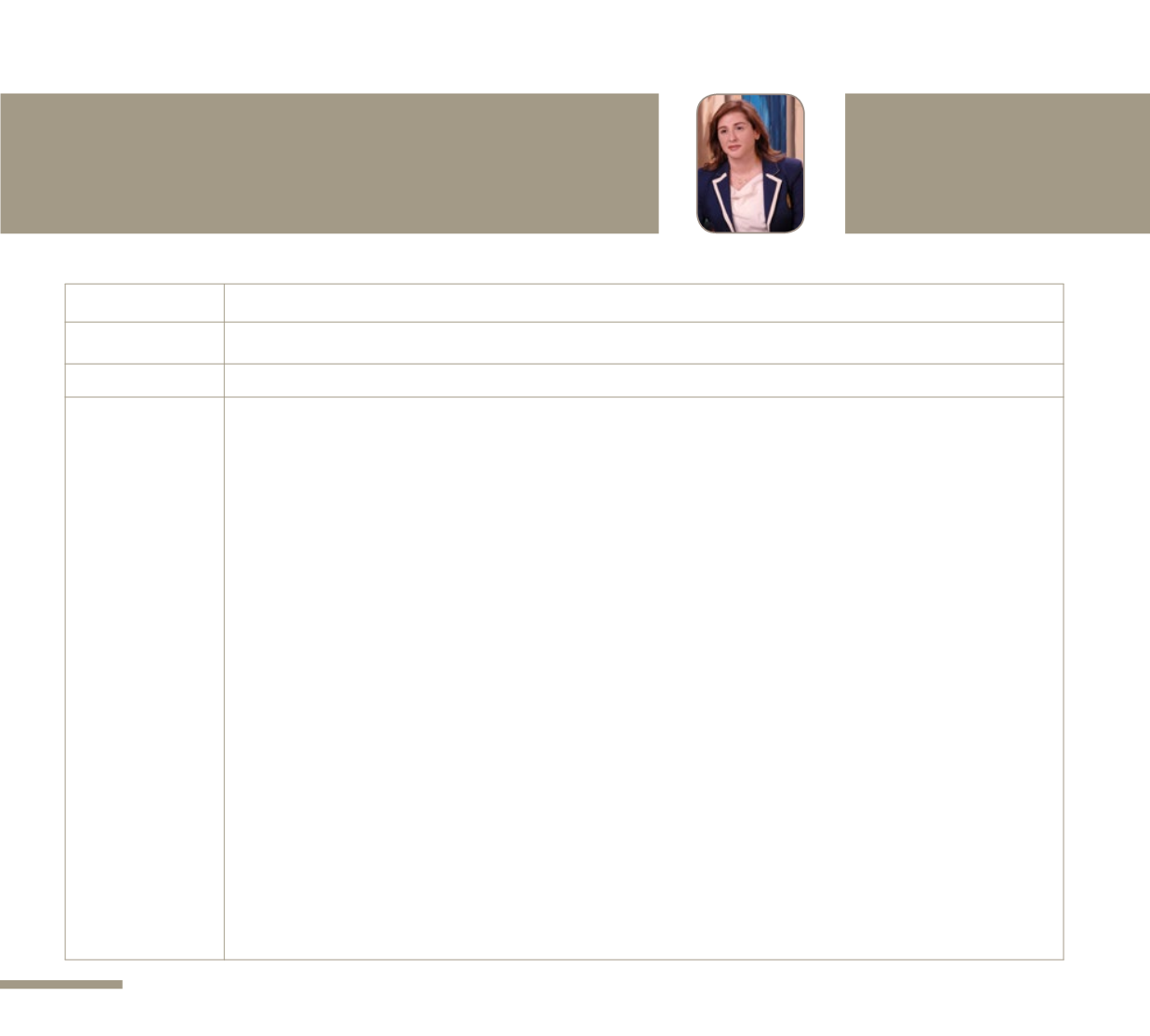
8
EILEEN MARIE HANNA
Department of Information Systems and Security
College of Information Technology
Title
Mining Biological Networks towards Protein-Complex Detection and Gene-Disease Association
Faculty Advisor
Prof. Nazar Zaki
Defense Date
30 September 2015
Abstract
Large amounts of biological data are continuously generated nowadays, thanks to the advancements of
high-throughput experimental techniques. Mining valuable knowledge from such data still motivates the
design of suitable computational methods, to complement the experimental work which is often restricted
by considerable time and cost requirements. Protein complexes, or groups of interacting proteins, are
key players in most cellular events. The identification of complexes not only allows to better understand
normal biological processes but also to uncover disease-triggering malfunctions. Ultimately, findings in this
research branch can highly enhance the design of effective medical treatments. The aim of this research
is to detect protein complexes in protein-protein interaction networks and to associate the detected
entities to diseases. The work is divided into three main objectives: first, develop a suitable method for
the identification of protein complexes in static interaction networks; second, model the dynamic aspect
of protein interaction networks and detect complexes accordingly; and third, design a learning model
to link proteins, and subsequently protein complexes, to diseases. In response to these objectives, we
present, ProRank+, a novel complex-detection approach based on a ranking algorithm and a merging
procedure. Then, we introduce DyCluster, which uses gene expression data, to model the dynamics of
the interaction networks, and we adapt the detection algorithm accordingly. Finally, we integrate network
topology attributes and several biological features of proteins to form a classification model for gene-
disease association. The reliability of the proposed methods is supported by various experimental studies
conducted to compare them with existing approaches. ProRank+ detects more protein complexes than
other state-of-the-art methods. DyCluster goes a step further and achieves a better performance than
similar techniques. Then, our learning model shows that combining topological and biological features can
greatly enhance the gene-disease association process. Finally, we present a comprehensive case study of
breast cancer in which we pinpoint disease genes using our learning model; and we subsequently detect
favorable groupings of those genes in a protein interaction network using the ProRank+ algorithm.
Dissertation