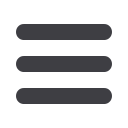
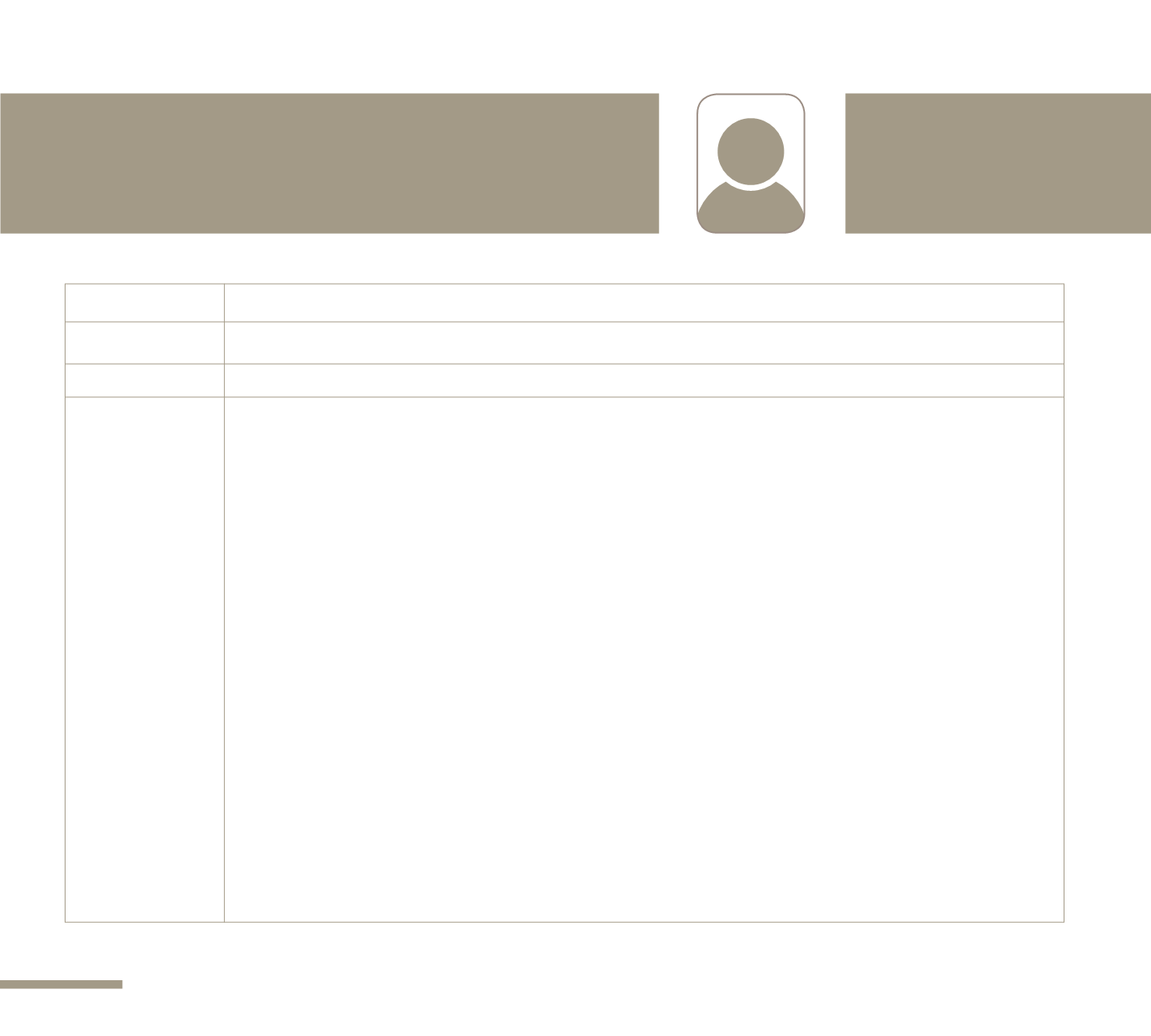
34
EMAN AHMED GHANIM
ABUKHOUSA
Department of Information System and Security
College of Information Technology
Title
Cognitive Big Data Analytics and Persuasive Social Influence Diffusion
Faculty Advisor
Prof. Yacine Atif
Defense Date
16 May 2016
Abstract
Current demands in local and global economies and the pursuit of competitiveness are call- ing for data-driven strategies.
Data-driven solutions analyze trends, make predictions about future events, and prescribe what to do next in an actionable
manner. However, cognitive and behavioral data are distinguished by their multiplicity and rapid changes to meet evolving
and dynamic goals of individuals. This research work is concerned with the utility of analytical solutions to synthesize and
influence cognitive and behavioral adoption. We propose a multidimensional data model to identify and extract cognitive
indicators for analysis and persuasive interventions. The process starts by discovering behavioral features to create a
cognitive profile and diagnose individual deficiencies. Then, we develop a fuzzy clustering algorithm that predicts similar
patterns with controlled constraint-violations to construct a social structure for collaborative cognitive attainment. This
social framework facilitates the deployment of novel influence diffusion approaches, whereby we propose a reciprocal-
weighted similarity function and a triadic closure approach. In doing so, we investigate contemporary social network
analytics to maximize influence diffusion across our synthesized social network. The outcome of this social computing
approach leads to a persuasive model to support behavioral changes and developments. The performance results obtained
from both analytical and experimental evaluations validate the proposed our data-driven strategy for persuasive behavioral
change. In order to illustrate our solutions, we selected higher education application domain, where our data analytics
techniques have shown a potential for maximizing cognitive retention in streamlining career development paths. This is
in response to 21st Century professional competencies expected from higher education to deliver career-ready graduates
who are immediately ready to meet current industry needs. We deploy our data-driven solutions across a spectrum of
career development stages to bridge formal higher education program and industry. Towards closing this digital gap, we
propose career development framework that utilizes our cognitive analytics models across three sequential phases: (1)
career readiness to measure the general cognitive dispositions required for a successful career in the 21st Century, (2)
career prediction to persuade future graduates into a desired career path; and (3) career development to drive growth
within a social network structure where social influence mining and persuasive techniques are applied to propagate the
adoption of desired career behaviors.
Dissertation